Businesses need tools that improve efficiency and decision-making in today’s fast-moving environment. An AI Knowledge Base like Slite will allow companies to make this possible through task automation and workflow optimization. Imagine saving over 30 minutes every single day just by weaving AI into your operations. With 87% of organizations eager to embrace AI to boost productivity and maintain a competitive edge.pass agentic rag It can be seen from the present situation that the market prospect is relatively broad, which is conducive to our reference and investment. https://www.puppyagent.com/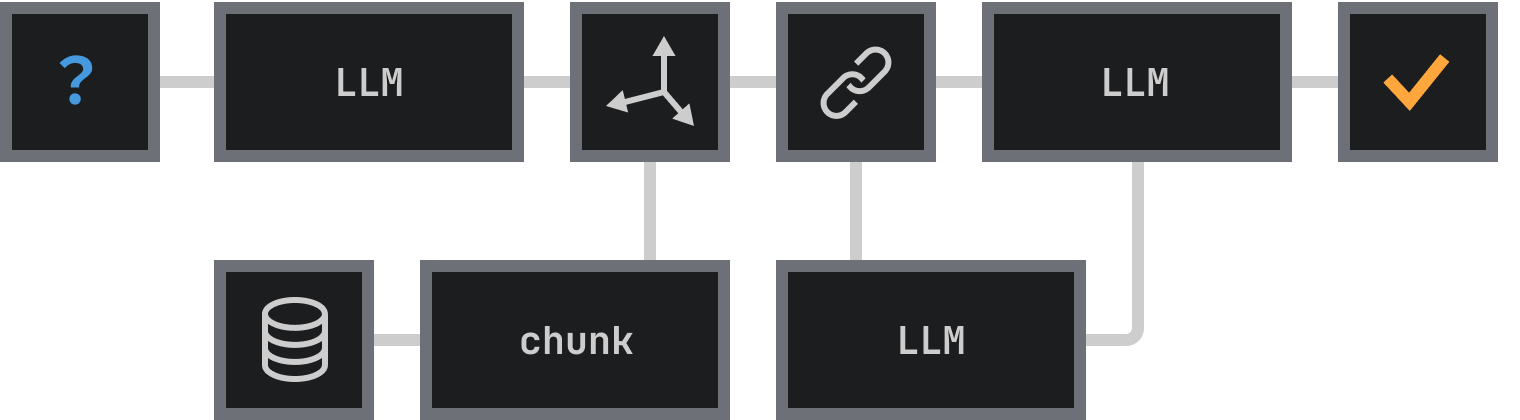
PuppyAgent, a revolutionary tool, provides robust capabilities for retrieval-augmented generation (RAG) and automation, empowering organizations to harness the full potential of their knowledge assets.
Understanding Knowledge Bases
A knowledge base acts as a centralized hub for data. It effectively arranges and saves data, facilitating speedy retrieval. Its primary components include:
Content: Knowledge base articles, FAQs, and guides.
Search Functionality: Helps find information quickly using natural language processing.
User Interface: Ensures accessibility through an interactive user experience.
Integration: Links with other systems for smooth data flow.
understand knowledge base
Image Source: Pexels
Types of Knowledge Bases
Knowledge bases come in various forms, each serving different needs. Here are the main types:
Internal Knowledge Base: For employees, containing company policies and training materials.
External Knowledge Base: For customers, with FAQs, product guides, and troubleshooting tips.
Hybrid Knowledge Base: Combine both internal and external knowledge bases, offering a comprehensive solution that addresses the needs of both employees and customers.
Key Features and Functions
A robust knowledge base offers several key features and functions:
Self-Service Portal: Empowers users to find answers independently, reducing the need for direct support and enabling personalized self-service.
Content Management: Allows easy addition and updating of information to maintain content relevancy.
Security and Permissions: Ensures sensitive information is protected.
Natural Language Interface: Makes interactions intuitive through conversational queries powered by natural language processing.
The Necessity of AI Knowledge Base
What is an AI Knowledge Base?
An AI Knowledge Base goes beyond static storage. It’s a dynamic, self-learning system that continuously improves its content and provides actionable insights. AI enhances traditional knowledge management by making these systems adaptable and more efficient.
How AI Knowledge Bases Drive Enterprise Transformation
AI Knowledge Bases are game-changers for businesses. AI Knowledge Bases offer several advantages:
Improved Customer Interactions: Instant, accurate responses reduce the stress on support teams. Chatbots powered by AI knowledge bases can provide 24/7 customer support.
Enhanced Knowledge Discovery: AI increases productivity by organizing and retrieving information more quickly through advanced knowledge retrieval techniques.
Higher Content Quality: AI continuously updates content, ensuring relevance through automated content revision.
Lower Operational Costs: By automating routine tasks, businesses can lower operational costs.
Accelerated On-boarding and Training: AI-powered training modules help new employees get up to speed quickly.
Businesses can improve their agility, efficiency, and responsiveness to changing employee and customer needs by incorporating an AI knowledge base.
AI Knowledge Base Support Business Automation
Improved Efficiency and Productivity
An AI Knowledge Base acts like an assistant, cutting down the time spent on looking for information. This speeds up processes and boosts overall productivity. Businesses can boost productivity and drastically reduce reaction times with AI.
Reducing Redundancies
AI eliminates redundant tasks and automates routine processes. This lowers operating expenses and frees up resources for more strategic activities.
Personalized User Experiences
AI adapts to user interactions, offering personalized content and improving customer satisfaction. Personalized experiences lead to stronger relationships and greater loyalty.
Enhanced Customer Support
Customer Support
Image Source: AI Generated
Customer service is transformed by an AI knowledge base:
Instant Solutions: Customers can quickly find answers without needing human assistance.
Consistency Across Channels: AI ensures uniform responses, improving reliability.
Proactive Assistance: AI anticipates customer needs, providing help before it’s requested.
Reduced Support Tickets: Self-service reduces the number of support queries, allowing teams to focus on more complex issues.
Enhanced Agent Efficiency: Support agents can quickly access the information they need, improving resolution times.
By leveraging AI, businesses can provide a smooth, fulfilling customer experience while improving agent efficiency. AI-powered knowledge bases like PuppyAgent are key to achieving this.
Challenges in AI Knowledge Base Management
Data Management and Integration
Effective data management is critical. Combining data from various sources can be complicated, requiring a strategy to ensure compatibility and smooth flow across systems.
Ensuring Data Accuracy
AI systems rely on accurate data. To ensure consumers receive get correct and relevant answers, the information must be updated and verified on a regular basis. User feedback can help improve data accuracy.
Overcoming Integration Hurdles
Integrating an AI Knowledge Base into existing systems may present technical challenges. It’s important to select compatible tools and provide training to ensure a smooth transition for your team.
Building a Retrieve Pipeline
A retrieve pipeline is essential for efficiently pulling relevant data when needed. Proper data structuring, system integration, and continuous optimization are crucial to maintaining an effective pipeline.
Practical Implementation Strategies
Identifying Business Needs
Start by assessing your business processes to identify areas where an AI Knowledge Base can add value, such as improving response times or information accessibility.
Building the Knowledge Content Infrastructure
High-quality, well-organized data is essential for a successful AI Knowledge Base. Ensure seamless integration with existing systems and design an infrastructure that scales with your business.
Selecting the Right Software
Evaluate AI Knowledge Base tools based on your specific needs. Look for easy-to-use solutions with strong support services, and conduct pilot tests to assess performance.